Introduction to Embodied Intelligence and Industrial AI
Embodied intelligence, a concept that integrates physical interaction and perception into AI systems, has emerged as a transformative force in various industries. It emphasizes the importance of physical presence and interaction in enhancing AI capabilities. In industrial scenarios, the development of physical AI systems often faces significant challenges, including high costs associated with data acquisition, model training, and deployment. These challenges are compounded by the need for specialized hardware and expertise, making it difficult for many industrial enterprises to fully leverage AI technologies.
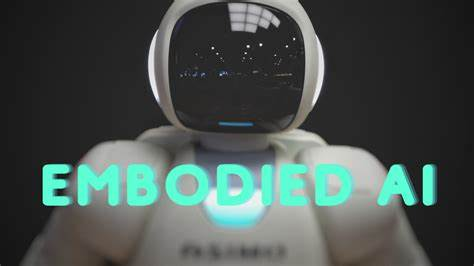
Overview of DeepSeek and NVIDIA’s Cosm
DeepSeek, a pioneering AI company, has made remarkable strides in developing advanced language models and AI technologies. Its models, such as DeepSeek-V2 and DeepSeek-R1, have demonstrated exceptional performance while significantly reducing training costs. These models employ innovative architectures like the Mixture-of-Experts (MoE) and Multi-Head Latent Attention (MLA), which enhance efficiency and reduce computational requirements. NVIDIA’s Cosm, on the other hand, is a powerful tool for generating synthetic data. Synthetic data can be tailored to specific industrial applications, providing a cost-effective alternative to large-scale real-world data collection.
Cost Reduction Strategies through Embodied Intelligence
One of the key strategies for reducing physical AI development costs in industrial scenarios is leveraging synthetic data. NVIDIA’s Cosm allows for the creation of high-quality synthetic datasets that can be used to train AI models. This approach eliminates the need for extensive real-world data collection, which can be time-consuming and expensive. By using synthetic data, industrial enterprises can train their models more efficiently and at a lower cost.
Embodied intelligence techniques further enhance the efficiency of AI models in industrial settings. By incorporating physical interaction and perception, these models can better understand and adapt to real-world environments. This reduces the need for extensive fine-tuning and optimization, which are often costly and time-consuming processes. Additionally, embodied intelligence enables more efficient use of computational resources, as models can focus on relevant information and ignore irrelevant data.
Case Study: Application of DeepSeek and Cosm in Industrial Settings
Several industrial enterprises have successfully applied DeepSeek’s models and NVIDIA’s Cosm to reduce development costs and improve performance. For example, a manufacturing company used synthetic data generated by Cosm to train a DeepSeek-based AI model for quality control. This approach significantly reduced the time and cost associated with data collection and model training. The AI model was able to accurately detect defects in products, improving overall quality control efficiency.
Another example is the application of embodied intelligence in robotics. By integrating physical sensors and actuators with AI models, robots can perform complex tasks more efficiently and accurately. This reduces the need for extensive programming and manual intervention, lowering operational costs. The use of synthetic data further enhances the training process, allowing robots to adapt to various industrial environments more quickly.
Future Prospects and Challenges
The future of embodied intelligence in industrial AI looks promising, with potential advancements in areas such as more efficient synthetic data generation and enhanced model adaptability. However, there are also challenges that need to be addressed. Ethical concerns, such as the potential impact on employment and privacy, must be carefully considered. Additionally, practical challenges like ensuring data quality and model reliability need to be overcome to fully realize the benefits of embodied intelligence in industrial applications.
Conclusion
In conclusion, embodied intelligence has the potential to significantly reduce the development costs of physical AI in industrial scenarios. By leveraging synthetic data and enhancing model efficiency, industrial enterprises can more effectively deploy AI technologies. The successful applications of DeepSeek’s models and NVIDIA’s Cosm demonstrate the practical benefits of these approaches. As the field of embodied intelligence continues to evolve, it is crucial to address ethical and practical challenges to ensure its sustainable and beneficial implementation in industrial settings.
Questions and Answers
- What is the main advantage of using synthetic data in industrial AI development?
- Synthetic data provides a cost-effective alternative to real-world data collection, reducing the time and resources required for model training.
- How does embodied intelligence enhance AI model efficiency in industrial scenarios?
- Embodied intelligence allows AI models to better understand and adapt to real-world environments by incorporating physical interaction and perception. This reduces the need for extensive fine-tuning and optimization.
- What are the key features of DeepSeek’s models that contribute to cost reduction?
- DeepSeek’s models, such as DeepSeek-V2 and DeepSeek-R1, employ innovative architectures like MoE and MLA. These architectures enhance model efficiency and reduce computational requirements, leading to lower training costs.
- Can you provide an example of how DeepSeek and NVIDIA’s Cosm have been used together in an industrial setting?
- A manufacturing company used synthetic data generated by NVIDIA’s Cosm to train a DeepSeek-based AI model for quality control. This approach significantly reduced the time and cost associated with data collection and model training, while improving overall quality control efficiency.
- What are the potential ethical concerns associated with the use of embodied intelligence in industrial AI?
- Ethical concerns include the potential impact on employment, as AI systems may replace certain jobs, and privacy issues related to data collection and usage.
- What are the future prospects for embodied intelligence in industrial AI?
- The future prospects include advancements in synthetic data generation and model adaptability. However, challenges such as ensuring data quality and model reliability need to be addressed to fully realize the benefits of embodied intelligence in industrial applications.