End-to-end AI represents a significant shift in how artificial intelligence is designed and implemented, particularly in the realm of embodied intelligence. This approach integrates perception, decision-making, and action into a unified framework, enabling more efficient and adaptive systems. This article explores the definition, prospects, and breakthroughs of end-to-end AI, as well as its differences from other AI development directions and the synergistic evolution of small and large models.
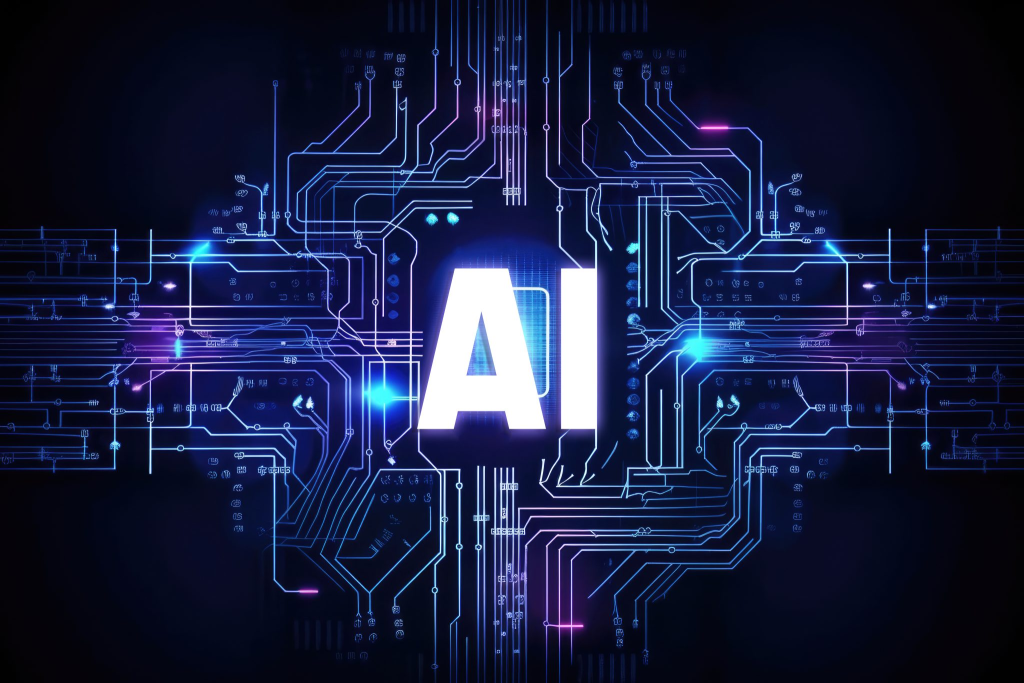
What is End-to-End AI?
End-to-end AI refers to a system where the input data (such as visual, auditory, or tactile signals) is directly processed to generate output actions, bypassing the need for manual feature extraction and task decomposition. This approach leverages deep learning techniques, particularly the Transformer architecture, to create a unified control strategy that can handle complex environments and tasks. The evolution of end-to-end AI has been driven by advancements in both software and hardware, with significant contributions from companies like NVIDIA, which has developed specialized AI chips to enhance processing speed and efficiency.
Prospects of End-to-End AI
The prospects of end-to-end AI are vast, with applications spanning across various sectors. In robotics, end-to-end AI enables robots to perform complex tasks with greater efficiency and adaptability. For instance, robots equipped with end-to-end AI systems can navigate unknown environments and complete tasks with minimal human intervention. In autonomous driving, end-to-end AI models are being used to improve decision-making and control, leading to safer and more efficient driving experiences. The future of end-to-end AI looks promising, with continued advancements expected to drive further integration into everyday life.
Comparison with Other AI Development Directions
End-to-end AI differs from traditional AI and modular systems in several key ways. Traditional AI often relies on handcrafted features and separate modules for different tasks, which can be inefficient and less adaptable. In contrast, end-to-end AI integrates all components into a single, unified system, allowing for more seamless operation and better performance in dynamic environments. This approach also simplifies the development process, as it reduces the need for extensive feature engineering and task-specific tuning.
Breakthroughs in End-to-End Models
Recent breakthroughs in end-to-End AI have been driven by advancements in Transformer architectures and reinforcement learning. These technologies enable AI systems to process large amounts of data more efficiently and make decisions based on complex patterns. Additionally, hardware improvements, such as specialized AI chips, have significantly enhanced the performance of end-to-end AI systems. These chips are designed to handle multiple types of input data simultaneously, allowing for real-time processing and faster response times.
Synergistic Evolution of Small and Large Models
The synergistic evolution of small and large models is a key trend in the development of end-to-end AI. Small models can be optimized for specific tasks, while large models provide a more general understanding of the environment. This approach allows for more efficient use of resources and better performance across a wide range of applications. For example, in autonomous driving, small models can handle specific tasks like lane detection, while large models can manage more complex decision-making processes.
Conclusion
End-to-end AI represents a significant advancement in the field of artificial intelligence, offering a more integrated and efficient approach to system design. With continued breakthroughs in technology and increasing applications across various sectors, end-to-end AI is poised to play a crucial role in the future of robotics, autonomous driving, and other fields. As we continue to explore the potential of this technology, the synergistic evolution of small and large models will be key to unlocking its full potential.
Questions and Answers
- What is the main difference between end-to-end AI and traditional AI systems?
- End-to-end AI integrates all components into a single, unified system, bypassing the need for manual feature extraction and task decomposition. This allows for more efficient and adaptive operation compared to traditional modular systems.
- What are the key applications of end-to-end AI?
- End-to-end AI is used in robotics for complex task execution, in autonomous driving for improved decision-making, and in various other fields where real-time processing and adaptability are crucial.
- How do hardware improvements impact end-to-end AI?
- Hardware advancements, such as specialized AI chips, significantly enhance the performance of end-to-end AI systems by enabling faster processing and real-time response to multiple types of input data.
- What role do small and large models play in the synergistic evolution of end-to-end AI?
- Small models are optimized for specific tasks, while large models provide a general understanding of the environment. This combination allows for more efficient resource use and better performance across a wide range of applications.
- What are the future prospects of end-to-end AI?
- The future of end-to-end AI looks promising, with continued advancements expected to drive further integration into everyday life. This includes applications in robotics, autonomous driving, and other sectors where adaptability and efficiency are crucial.
- What are some of the challenges in implementing end-to-end AI systems?
- Challenges include ensuring robustness and reliability in complex environments, managing computational resources efficiently, and addressing ethical and safety concerns related to autonomous systems.