In the rapidly evolving world of artificial intelligence, the cost of developing and deploying advanced models has always been a significant barrier to entry. However, DeepSeek, a Chinese AI startup, has emerged as a game-changer by offering powerful AI capabilities at a fraction of the cost associated with traditional models. This article delves into the training costs of DeepSeek, exploring how it achieves such remarkable cost efficiency and what this means for the future of AI.
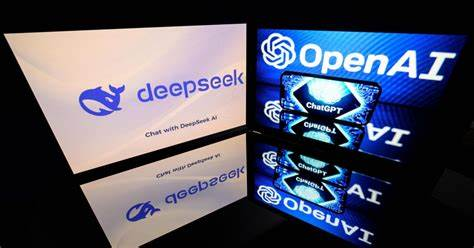
Training Cost Analysis
DeepSeek’s models have garnered attention for their impressive performance and remarkably low training costs. For instance, the DeepSeek-V3 model, which boasts 671 billion parameters, was reportedly trained for just $5.58 million over a period of two months. This is a stark contrast to models like Meta’s Llama 3 405B, which required 30.8 million GPU-hours and a significantly higher budget. The training of DeepSeek-V3 utilized 2048 GPUs, resulting in only 2.8 million GPU-hours, demonstrating that high performance does not necessarily demand massive investment and extensive training time.
When compared to other major AI models, DeepSeek’s cost efficiency becomes even more evident. For example, GPT-4’s training cost is estimated to be around $500 million. DeepSeek’s ability to achieve competitive results with such a low budget highlights its innovative approach to model development and training.
Pre-training Costs
Pre-training costs are another crucial aspect to consider. DeepSeek’s pre-training phase is optimized to be highly efficient, leveraging techniques like reinforcement learning to drive performance gains without the need for extensive supervised fine-tuning. This approach not only reduces the time and resources required for pre-training but also allows the model to develop more robust and flexible reasoning capabilities.
Factors Contributing to Cost Efficiency
Several factors contribute to DeepSeek’s cost efficiency. Firstly, its reliance on open-source technology allows for greater flexibility and customization, reducing the need for expensive proprietary solutions. The use of innovations like the Mixture-of-Experts (MoE) architecture, which selectively activates only a portion of the model’s parameters for each task, significantly improves computational efficiency. This selective activation reduces the overall hardware requirements and associated costs.
Additionally, strategic partnerships play a vital role in DeepSeek’s cost-effective approach. For example, its collaboration with AMD, utilizing AMD Instinct GPUs and open-source ROCM software, enables DeepSeek to train its models at remarkably low costs. This partnership challenges the industry’s reliance on high-end NVIDIA GPUs or Google TPUs, proving that efficient training can be achieved without the most expensive hardware.
Implications for the AI Industry
DeepSeek’s cost model has far-reaching implications for the AI industry. By offering powerful AI capabilities at a fraction of the cost, DeepSeek challenges the status quo dominated by proprietary models. Its open-source nature and low API pricing make advanced AI more accessible to a broader audience, including startups, researchers, and small businesses. This accessibility has the potential to democratize AI, fostering greater innovation and adoption across various sectors.
Conclusion
DeepSeek’s remarkable cost efficiency in training and pre-training its models sets a new standard in the AI landscape. By leveraging open-source technology, innovative model architectures, and strategic partnerships, DeepSeek has managed to achieve high performance at a fraction of the cost associated with traditional models. As the AI industry continues to evolve, DeepSeek’s cost-effective approach could pave the way for a more inclusive and innovative future, where advanced AI capabilities are no longer limited to well-funded organizations.
相关问题及解答
- How does DeepSeek manage to keep its training costs so low?
- DeepSeek achieves low training costs through a combination of open-source technology, innovations like the Mixture-of-Experts (MoE) architecture, and strategic partnerships with companies like AMD.
- Is DeepSeek’s performance compromised due to its low training costs?
- No, DeepSeek’s performance is not compromised. In fact, its models have shown competitive performance in various benchmarks, often outperforming other top models despite the lower training costs.
- How does DeepSeek’s cost model benefit small businesses and startups?
- DeepSeek’s low-cost model makes advanced AI accessible to small businesses and startups, allowing them to leverage powerful AI capabilities without the prohibitive costs associated with other models.
- What are the key innovations in DeepSeek’s training approach?
- DeepSeek uses a large-scale reinforcement learning approach, reward engineering, and efficient knowledge distillation techniques to achieve high performance with lower training costs.
- How does DeepSeek’s open-source policy impact its cost efficiency?
- DeepSeek’s open-source policy allows for greater flexibility and customization, reducing the need for expensive proprietary solutions. This approach helps in lowering both development and deployment costs.
- What is the potential impact of DeepSeek’s cost model on the AI industry?
- DeepSeek’s cost model challenges the status quo by making advanced AI more accessible and affordable. This could lead to greater innovation and adoption across various sectors, including startups and small businesses.