In the rapidly evolving landscape of artificial intelligence, DeepSeek has emerged as a formidable contender, challenging the dominance of established models like GPT-4o, Claude-3.5-Sonnet, Qwen2.5, and Llama-3.1. This article delves into a comprehensive comparison of DeepSeek’s performance against these global top models, exploring key metrics, cost efficiency, and specific use cases.
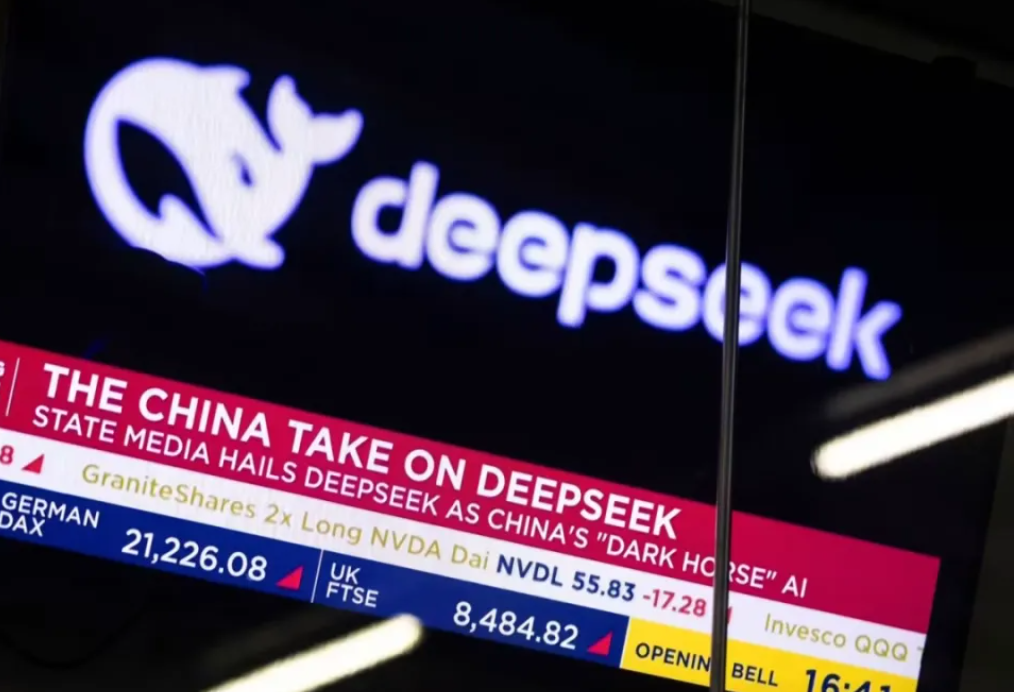
DeepSeek’s Performance Overview
DeepSeek’s unique approach to AI development sets it apart from its competitors. Utilizing a Mixture-of-Experts (MoE) architecture, DeepSeek-R1 boasts 671 billion parameters, with only 37 billion active at any given time. This design not only enhances computational efficiency but also reduces operational costs significantly. In benchmark evaluations, DeepSeek has demonstrated state-of-the-art performance across a wide range of tasks, particularly excelling in reasoning, coding, and long-context understanding.
Comparison with GPT-4o
When compared to OpenAI’s GPT-4o, DeepSeek’s performance is notably competitive. In logical problem-solving and math computations, DeepSeek has outperformed GPT-4o in several third-party benchmarks. Moreover, DeepSeek’s cost efficiency is a major advantage. While GPT-4o’s operational costs are estimated to be in the hundreds of millions of dollars, DeepSeek’s training budget for its R1 model was a mere $5.6 million. This cost advantage, coupled with its strong performance in technical tasks, makes DeepSeek a compelling alternative for enterprises and developers seeking a more affordable yet powerful AI solution.
Comparison with Claude-3.5-Sonnet
Claude-3.5-Sonnet by Anthropic is another leading model in the AI space. In terms of performance, Claude-3.5-Sonnet has shown significant improvements in coding and tool use tasks. However, DeepSeek-V3 has managed to close the gap in many areas. For instance, in the MMLU-Pro benchmark, DeepSeek-V3 closely trails Claude-3.5-Sonnet, demonstrating its robust capabilities in educational knowledge tasks. Additionally, DeepSeek’s open-source nature provides greater flexibility and adaptability for developers, unlike Claude-3.5-Sonnet’s closed-source approach.
Comparison with Qwen2.5
Qwen2.5 is known for its strong performance in coding and structured data understanding. While Qwen2.5 has shown impressive results in benchmarks such as HumanEval and MATH, DeepSeek-V3 has also made significant strides in these areas. For example, DeepSeek-V3 achieved a score of 49.5 in DS-Arena-Code, showcasing its coding capabilities. In terms of cost, DeepSeek’s pricing structure is highly competitive, with input and output token costs significantly lower than those of Qwen2.5. This cost-effectiveness, combined with its robust performance, makes DeepSeek an attractive option for developers and businesses.
Comparison with Llama-3.1
Llama-3.1, developed by Meta, is a dense architecture model with 405 billion parameters. While it offers powerful expressiveness, its dense parameter utilization results in higher computational demands and costs. In contrast, DeepSeek’s sparse MoE architecture allows it to achieve comparable performance at a fraction of the cost. For instance, the training cost for Llama-3.1 can range from $92.4 million to $123.2 million, whereas DeepSeek-V3’s training cost was only $5.6 million. This cost-to-performance ratio makes DeepSeek a more sustainable and scalable option for large-scale AI deployment.
Conclusion
DeepSeek’s innovative approach to AI development has positioned it as a strong competitor in the global AI market. Its Mixture-of-Experts architecture enables high performance while maintaining cost efficiency, making it an attractive choice for a wide range of applications. While there are areas where DeepSeek can further improve, such as in conversational AI and factual knowledge tasks, its strengths in reasoning, coding, and long-context understanding are undeniable. As DeepSeek continues to evolve and expand its capabilities, it holds great potential to shape the future of AI development.
Frequently Asked Questions
- How does DeepSeek’s performance in reasoning tasks compare to GPT-4o?
- DeepSeek has shown superior performance in logical problem-solving and math computations, outperforming GPT-4o in several third-party benchmarks.
- What are the cost advantages of using DeepSeek over other models like Llama-3.1?
- DeepSeek’s training cost is significantly lower than that of Llama-3.1. While Llama-3.1’s training costs can range from $92.4 million to $123.2 million, DeepSeek-V3 was trained for only $5.6 million.
- Does DeepSeek’s open-source nature impact its performance compared to closed-source models like Claude-3.5-Sonnet?
- DeepSeek’s open-source approach does not compromise its performance. In fact, it allows for greater flexibility and community-driven improvements, making it competitive with closed-source models like Claude-3.5-Sonnet.
- How does DeepSeek handle long-context tasks compared to GPT-4o?
- DeepSeek-V3 demonstrates strong capabilities in long-context understanding, achieving high F1 scores in benchmarks like DROP and FRAMES. It closely trails GPT-4o in these tasks while outperforming other models.
- What are the key strengths of DeepSeek in coding tasks?
- DeepSeek excels in coding tasks, particularly in algorithmic tasks like HumanEval and LiveCodeBench. Its advanced knowledge distillation technique enhances its code generation and problem-solving capabilities.
- Is DeepSeek suitable for conversational AI applications like Claude-3.5-Sonnet?
- While DeepSeek is highly effective in technical tasks, it may not be as optimized for conversational AI applications as Claude-3.5-Sonnet. However, its open-source nature allows for further customization and improvement in this area.