Artificial intelligence has witnessed a surge of groundbreaking advancements in recent years, with large language models (LLMs) taking center stage. Among these, DeepSeek, a Chinese AI startup, has garnered significant attention for its innovative approach and remarkable performance. This article delves into the technical architecture of DeepSeek and explores the core differences between DeepSeek and other prominent large models such as GPT-4 and Claude.
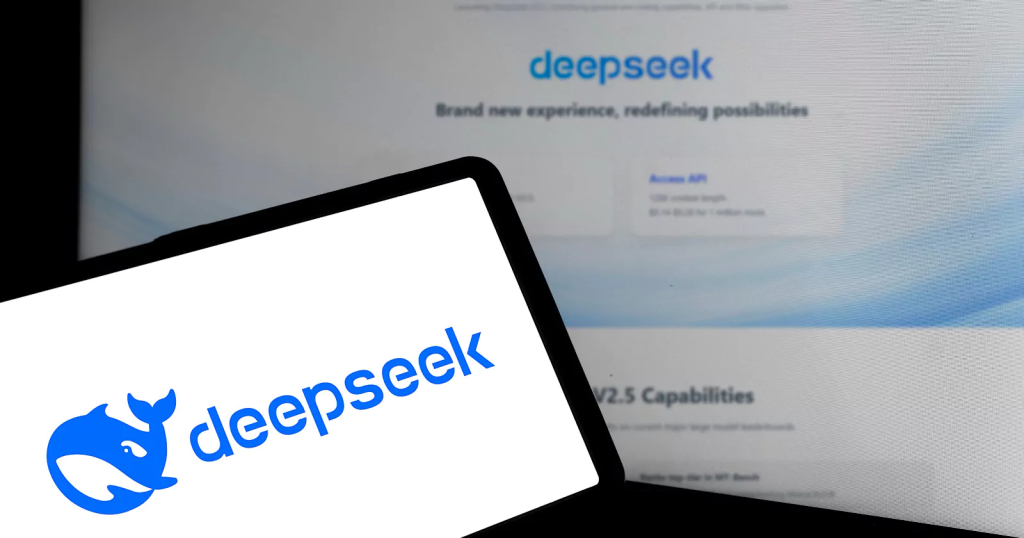
DeepSeek, founded in 2023, is on a mission to advance artificial general intelligence (AGI) through open-source research and development. The company’s technical architecture is a key factor behind its success. DeepSeek’s models, such as DeepSeek-V3 and DeepSeek-R1, employ a Mixture-of-Experts (MoE) system, which selectively activates only a portion of the parameters for each task. This approach significantly improves efficiency and reduces computational costs while maintaining top-tier performance. Additionally, DeepSeek utilizes a Multi-head Latent Attention Transformer in its architecture. The training process is enhanced by Group Relative Policy Optimization (GRPO), a reinforcement learning technique that allows the AI to refine its reasoning more effectively.
When comparing DeepSeek with GPT-4, several key differences emerge. In terms of architecture, DeepSeek’s MoE system with a Multi-head Latent Attention Transformer sets it apart from GPT-4’s architecture. The training approach also varies, with DeepSeek employing GRPO, whereas GPT-4 relies on a different set of training techniques. Performance-wise, DeepSeek has demonstrated competitive capabilities, with models like DeepSeek-V3 matching or even surpassing GPT-4 in certain benchmarks. One of the most notable differences is the open-source nature of DeepSeek, which contrasts with GPT-4’s closed-source model. This openness allows for greater transparency, community involvement, and the potential for further customization and innovation.
Similarly, when comparing DeepSeek with Claude, distinct differences can be observed. Claude’s architecture and training methods differ from DeepSeek’s MoE system and GRPO approach. In terms of performance, both models have their strengths, with DeepSeek showing superior performance in certain areas such as math and reasoning benchmarks. However, Claude maintains a slight edge in coding tasks according to some benchmarks. The open-source nature of DeepSeek is again a significant point of differentiation from Claude, which is also a closed-source model.
The core differences in technical architecture between DeepSeek and other large models have several important implications. Firstly, DeepSeek’s approach leads to greater cost-efficiency, as demonstrated by the relatively low training costs of its models. This cost-effectiveness makes advanced AI technology more accessible to a broader audience, including developers, businesses, and educational institutions. The open-source nature of DeepSeek fosters a community-driven approach, encouraging collaboration, innovation, and the potential for rapid advancements in the field.
In conclusion, DeepSeek’s technical architecture, characterized by its Mixture-of-Experts system, Multi-head Latent Attention Transformer, and Group Relative Policy Optimization, sets it apart from other large models like GPT-4 and Claude. The open-source nature of DeepSeek further differentiates it, offering transparency, accessibility, and the potential for community-driven development. As DeepSeek continues to push the boundaries of AI, its innovative approach and commitment to open-source development are likely to have a lasting impact on the industry.
暂无评论内容