AI-Enhanced Resilience of Smart Grids: Navigating Extreme Weather and Carbon Footprint Tracking
In the face of increasing extreme weather events and the growing demand for sustainable energy solutions, the integration of Artificial Intelligence (AI) into smart grid systems has become a critical development in modern energy management. AI not only enhances the efficiency and reliability of smart grids but also plays a vital role in ensuring their resilience during extreme weather conditions. Additionally, AI-driven carbon footprint tracking offers a powerful tool for monitoring and reducing environmental impact. This article explores how AI is transforming smart grid resilience, the detailed concept of AI-driven carbon footprint tracking, and the latest advancements in renewable energy prediction.
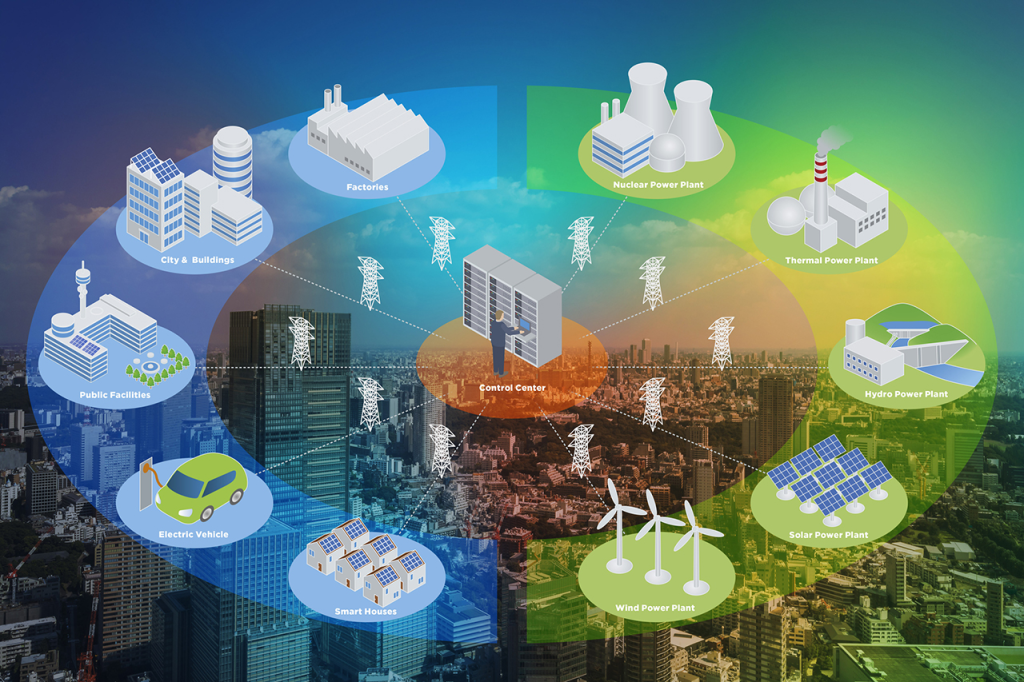
AI-Driven Resilience in Smart Grids
The concept of resilience in smart grids refers to the ability of the electrical grid to withstand and recover quickly from disruptions caused by extreme weather events such as hurricanes, floods, and heatwaves. AI enhances this resilience by providing real-time monitoring, predictive analytics, and automated response mechanisms. For example, AI algorithms can analyze vast amounts of data from sensors and weather forecasts to predict potential outages and reroute power to minimize disruptions.
Case studies have shown significant benefits of AI in enhancing grid resilience. In regions prone to extreme weather, AI-driven smart grids have demonstrated their ability to quickly restore power after outages. For instance, during a severe cold weather event in Texas, AI systems were able to optimize energy distribution and prevent widespread blackouts. Similarly, AI has been used to manage power supply during extreme heatwaves in California, ensuring that critical infrastructure remains operational.
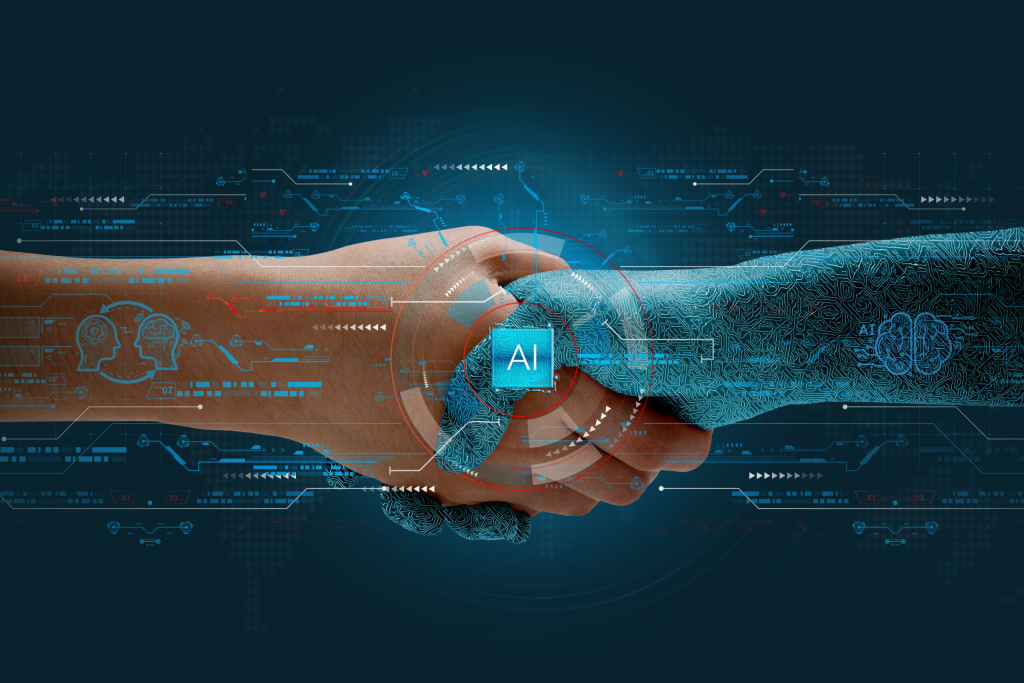
AI-Driven Carbon Footprint Tracking
AI-driven carbon footprint tracking involves the use of advanced analytics and machine learning algorithms to monitor and manage carbon emissions across various sectors of the energy system. This technology allows for real-time monitoring of energy consumption patterns and provides insights into areas where emissions can be reduced. For example, AI can analyze the energy efficiency of buildings, industrial processes, and transportation systems, identifying opportunities for optimization and sustainability improvements.
The benefits of AI-driven carbon footprint tracking are numerous. It provides transparency and accountability, enabling organizations to set and meet their sustainability goals. Additionally, AI can help policymakers make informed decisions by providing detailed data on carbon emissions and potential reduction strategies.
Recent Advancements in Renewable Energy Prediction
The latest advancements in AI for renewable energy prediction have significantly improved the accuracy and reliability of forecasting solar and wind power generation. AI algorithms can now analyze complex weather patterns, satellite imagery, and historical data to provide precise predictions of renewable energy output. This allows energy providers to better integrate renewable sources into the grid, reducing reliance on fossil fuels and enhancing overall grid stability.
For example, AI models can predict solar power generation based on cloud cover, temperature, and other meteorological factors. Similarly, wind power predictions can be made by analyzing wind speed, direction, and atmospheric conditions. These advancements have a profound impact on grid management, enabling more efficient use of renewable energy and reducing the need for backup power sources.
Challenges and Future Directions
Despite the significant benefits of AI in enhancing smart grid resilience and carbon footprint tracking, several challenges remain. One of the primary challenges is the high computational demand of AI systems, which can lead to increased energy consumption and carbon emissions from data centers. Additionally, the integration of AI into existing grid infrastructure requires significant investment and technical expertise.
Future advancements in AI for energy management will focus on developing more efficient algorithms and hardware to reduce computational demands. Additionally, the development of green AI solutions, which prioritize sustainability in AI operations, will be crucial for minimizing environmental impact. Other potential solutions include the use of edge computing and quantum computing to enhance the efficiency and scalability of AI systems.
Conclusion
AI has emerged as a transformative force in enhancing the resilience of smart grids during extreme weather conditions and in driving sustainable energy management through carbon footprint tracking and renewable energy prediction. By leveraging advanced analytics and machine learning, AI provides real-time monitoring, predictive capabilities, and optimization strategies that significantly improve grid reliability and environmental performance. As technology continues to advance, AI will play an increasingly important role in shaping a more resilient and sustainable energy future.
FAQs
- How does AI enhance the resilience of smart grids during extreme weather conditions? AI enhances grid resilience by providing real-time monitoring, predictive analytics, and automated response mechanisms. It can predict potential outages and reroute power to minimize disruptions.
- What is AI-driven carbon footprint tracking? AI-driven carbon footprint tracking uses advanced analytics and machine learning to monitor and manage carbon emissions across various sectors. It provides real-time data on energy consumption and identifies opportunities for optimization.
- What are the latest advancements in renewable energy prediction using AI? Recent advancements include the use of AI algorithms to analyze complex weather patterns and historical data, providing precise predictions of renewable energy output. This enhances grid stability and reduces reliance on fossil fuels.
- What challenges are associated with implementing AI for grid resilience and carbon tracking? Challenges include high computational demands, integration with existing infrastructure, and the need for significant investment and technical expertise.
- How can AI help reduce carbon emissions from data centers? AI can optimize data center operations to reduce energy consumption, improve cooling efficiency, and source renewable energy. Additionally, developing green AI solutions can minimize the environmental impact of AI systems.
- What is the future direction for AI in energy management? Future advancements will focus on developing more efficient algorithms and hardware, leveraging green AI solutions, and exploring new computing architectures like edge and quantum computing.