In the era of rapid technological advancement, the integration of Artificial Intelligence (AI) into energy management systems has emerged as a transformative force. AI’s ability to optimize energy distribution, predict renewable energy output, and track carbon footprints holds the promise of a more sustainable and efficient energy future. However, this progress comes with challenges, particularly in balancing the computational power required by AI systems and the environmental goals of reducing carbon emissions. This article explores the multifaceted role of AI in energy management, focusing on smart grid optimization, renewable energy prediction, and carbon footprint tracking, while also addressing the critical issue of balancing AI’s computational needs with sustainability.
AI in Smart Grid Optimization
A smart grid is an advanced electrical grid that incorporates digital technology to monitor and control the flow of electricity in real-time. The integration of AI into smart grids enhances their functionality by enabling predictive analytics, demand response management, and fault detection. AI algorithms can analyze vast amounts of data from sensors and meters to optimize energy distribution, reducing waste and improving efficiency. For instance, AI can predict peak demand periods and adjust energy allocation accordingly, ensuring that supply meets demand without overloading the grid.
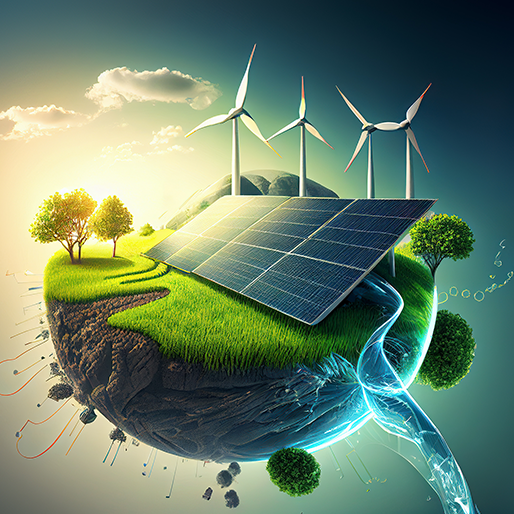
One notable case study is the resilience of smart grids in extreme weather conditions. In regions prone to hurricanes, AI-driven smart grids have demonstrated their ability to quickly restore power after outages. By analyzing weather data and grid performance in real-time, AI systems can reroute power and prioritize repairs, minimizing downtime and enhancing grid resilience. This capability is crucial as climate change increases the frequency and intensity of extreme weather events.
Predictive Analytics for Renewable Energy
Renewable energy sources, such as wind and solar power, are intermittent by nature. The unpredictability of these sources poses challenges for energy grid reliability. However, AI has proven to be a game-changer in this area. By analyzing historical weather data, satellite imagery, and real-time sensor data, AI algorithms can accurately predict the output of wind turbines and solar panels. These predictions allow energy providers to better manage the integration of renewable energy into the grid, ensuring a stable supply of electricity.
For example, AI models can forecast solar power generation based on cloud cover, temperature, and other meteorological factors. Similarly, wind power predictions can be made by analyzing wind speed, direction, and atmospheric conditions. These predictive capabilities not only improve grid reliability but also reduce the need for backup fossil fuel power plants, contributing to a more sustainable energy system.
However, achieving accurate predictions is not without challenges. The complexity of weather patterns and the variability of renewable energy sources require sophisticated AI models and large amounts of data. Additionally, the integration of these predictions into grid management systems requires seamless communication and coordination between different stakeholders. Despite these challenges, the benefits of AI-driven renewable energy prediction far outweigh the difficulties, paving the way for a more sustainable energy future.
AI-Driven Carbon Footprint Tracking
As the world increasingly focuses on reducing carbon emissions, the ability to accurately track and monitor carbon footprints has become essential. AI-driven carbon footprint tracking systems provide a powerful tool for measuring and managing emissions across various sectors. By analyzing data from energy consumption, transportation, and industrial processes, AI algorithms can identify areas of high carbon emissions and suggest strategies for reduction.
For example, AI can analyze the energy consumption patterns of buildings and recommend energy-efficient upgrades, such as better insulation or more efficient HVAC systems. In the transportation sector, AI can optimize routes for delivery trucks, reducing fuel consumption and emissions. These applications of AI not only help organizations meet their sustainability goals but also provide valuable insights for policymakers and regulators.
Real-world applications of AI-driven carbon footprint tracking have shown significant benefits. Companies that implement these systems often report reduced energy costs and improved environmental performance. Moreover, the transparency provided by AI-driven tracking enables better decision-making and accountability, driving a culture of sustainability within organizations and across industries.
Balancing AI’s Computational Needs with Sustainability
While AI offers numerous benefits for energy management, its increasing computational demands pose a challenge for sustainability. Data centers, which are the backbone of AI operations, consume vast amounts of energy and contribute to carbon emissions. As AI systems become more complex and data-intensive, the energy consumption of data centers is projected to grow significantly. This growth in carbon emissions threatens to undermine the environmental benefits that AI brings to energy management.
To address this challenge, several strategies can be employed. First, improving the energy efficiency of data centers through advanced cooling technologies and renewable energy sourcing can reduce their carbon footprint. Second, optimizing AI algorithms to run more efficiently on existing hardware can minimize energy consumption without sacrificing performance. Finally, exploring alternative computing architectures, such as edge computing and quantum computing, can provide more sustainable solutions for AI operations.
Looking to the future, the development of green AI is a promising direction. Green AI focuses on designing AI systems that are both powerful and energy-efficient. By leveraging innovations in hardware design, algorithm optimization, and renewable energy integration, green AI aims to maximize the benefits of AI while minimizing its environmental impact. This approach is crucial for ensuring that AI remains a sustainable solution for energy management in the long term.
Conclusion
The integration of AI into energy management systems represents a significant step forward in the quest for sustainable and efficient energy use. Through smart grid optimization, renewable energy prediction, and carbon footprint tracking, AI has the potential to revolutionize the way we manage and consume energy. However, the challenge of balancing AI’s computational needs with sustainability goals cannot be overlooked. By employing strategies to reduce the carbon footprint of data centers and developing green AI solutions, we can harness the full potential of AI while preserving our environment for future generations.
FAQs
- How does AI improve the efficiency of smart grids? AI enhances smart grid efficiency by analyzing real-time data to optimize energy distribution, predict demand, and detect faults. This reduces waste and ensures a stable supply of electricity.
- What role does AI play in renewable energy prediction? AI analyzes historical and real-time data to forecast the output of renewable energy sources like wind and solar. Accurate predictions help integrate these intermittent sources into the grid more effectively.
- Why is carbon footprint tracking important? Carbon footprint tracking is crucial for identifying high-emission areas and implementing strategies to reduce emissions. It provides transparency and accountability, driving sustainability efforts.
- How can we reduce the carbon footprint of data centers? Strategies include improving data center energy efficiency through advanced cooling technologies, sourcing renewable energy, and optimizing AI algorithms to run more efficiently.
- What is green AI, and why is it important? Green AI focuses on developing energy-efficient AI systems that minimize carbon emissions. It is essential for ensuring that AI remains a sustainable solution for energy management.
- Can AI help in extreme weather resilience for smart grids? Yes, AI-driven smart grids can quickly restore power after outages by analyzing weather data and rerouting energy. This enhances grid resilience in extreme weather conditions.
References
- “The Role of AI in Smart Grids: Enhancing Resilience and Efficiency,” IEEE Transactions on Smart Grid, Link
- “AI for Renewable Energy Forecasting: Challenges and Opportunities,” Renewable and Sustainable Energy Reviews, Link
- “Carbon Footprint Tracking with AI: A Path to Sustainability,” Environmental Research Letters, Link
- “Green AI: Towards Sustainable Machine Learning,” Nature Machine Intelligence, Link