1. Definition and Background of Autonomous Driving
Autonomous driving, often referred to as self-driving or driverless technology, involves the use of advanced sensors, algorithms, and artificial intelligence to enable vehicles to navigate and operate without human intervention. This technology has the potential to revolutionize transportation by increasing safety, reducing traffic congestion, and enhancing mobility for people who are unable to drive.
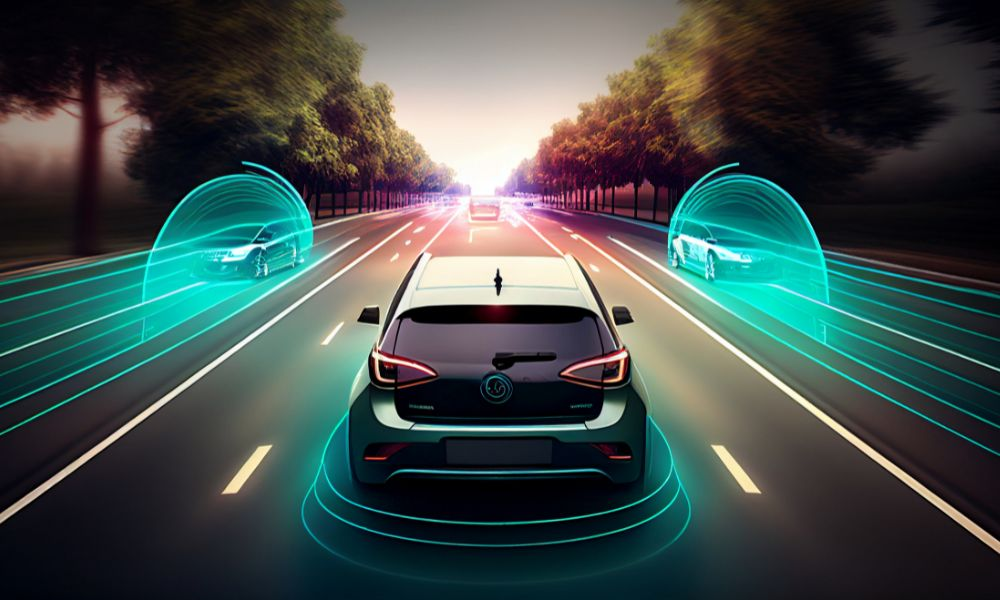
The development of autonomous driving is driven by several factors, including advancements in AI, the increasing demand for safer and more efficient transportation, and the growing interest from both automotive manufacturers and tech companies in exploring new mobility solutions.
2. The Current State of Autonomous Driving
Technical Levels and Applications
Autonomous driving technologies are categorized into different levels, ranging from Level 0 (no automation) to Level 5 (full automation). Currently, Level 2 (partial automation) and Level 2+ (advanced driver-assistance systems) are the most widely used, offering features such as adaptive cruise control and lane-keeping assistance. However, the industry is making significant strides towards higher levels of automation, with some companies already testing Level 3 and Level 4 vehicles.
Market Size and Commercialization
The global market for autonomous driving is experiencing rapid growth. In China, the market size is projected to reach 383.2 billion yuan in 2024, indicating a substantial potential for expansion. The commercialization of autonomous driving is also accelerating, with applications in various sectors such as ride-hailing services, logistics, and public transportation.
3. Future Trends in Autonomous Driving
End-to-End Autonomous Driving
End-to-end autonomous driving, which uses a single neural network to process inputs from sensors and generate driving decisions, is gaining traction. This approach simplifies the system architecture and improves efficiency. Tesla’s FSD V12 is a notable example, demonstrating significant advancements in autonomous driving capabilities.
Multimodal Fusion and AI Models
The integration of multimodal data (such as visual, auditory, and textual information) with large AI models is another emerging trend. This fusion enhances the vehicle’s ability to understand and interact with its environment, making driving decisions more accurate and reliable.
Expansion of Autonomous Services
Autonomous taxi services, known as Robotaxi, are expanding globally. Companies like Waymo in the US and Baidu Apollo in China are leading the way, offering driverless taxi services in multiple cities. These services are expected to grow significantly in the coming years, transforming urban transportation.
4. The Relationship Between AI Models and Autonomous Driving
AI models, particularly large language models (LLMs), are becoming a core component of autonomous driving systems. They are used in perception, decision-making, and control modules to improve object recognition, path planning, and real-time environment understanding. For example, Wayve’s Lingo-1 model enables vehicles to understand and execute natural language instructions, making interactions more intuitive.
5. Applications of Current Popular Technologies
Tesla’s FSD V12
Tesla’s Full Self-Driving (FSD) V12 represents a significant leap in end-to-end autonomous driving. It leverages advanced neural networks and data-driven approaches to achieve longer stretches of autonomous driving in complex urban environments.
Waymo and XPeng’s Robotaxi Operations
Waymo has been a pioneer in Robotaxi services, operating driverless taxis in several US cities. XPeng Motors is also making progress in this area, aiming to provide autonomous ride-hailing services in China. These companies are focusing on improving the safety and reliability of their systems through continuous testing and optimization.
VLM (Visual-Language Model) Integration
Visual-Language Models (VLMs) are being explored to enhance the interaction between vehicles and humans. By combining visual data with natural language processing, VLMs can enable vehicles to better understand and respond to human commands and gestures, making autonomous driving more user-friendly.
6. Conclusion and Outlook
The future of autonomous driving is promising, with continuous advancements in technology and increasing commercial applications. However, challenges such as ensuring safety, regulatory approvals, and public acceptance remain. The integration of AI models and multimodal data will play a crucial role in overcoming these challenges and realizing the full potential of autonomous driving.
Hot Questions and Answers
- What are the main challenges facing autonomous driving today?
- The primary challenges include ensuring the safety and reliability of autonomous systems, addressing regulatory and legal issues, and gaining public trust. Additionally, technical challenges such as improving sensor accuracy and handling complex urban environments remain.
- How does end-to-end autonomous driving differ from traditional approaches?
- End-to-end autonomous driving uses a single neural network to process inputs and generate outputs directly, eliminating the need for separate modules for perception, planning, and control. This approach simplifies the system and improves efficiency.
- What role do AI models play in improving autonomous driving?
- AI models enhance the vehicle’s ability to perceive its environment, make decisions, and interact with humans. They enable more accurate object recognition, path planning, and natural language understanding, which are crucial for safe and efficient autonomous driving.
- Why are Robotaxi services important for the future of transportation?
- Robotaxi services have the potential to transform urban transportation by providing a more efficient, sustainable, and accessible mode of travel. They can reduce the need for private car ownership, decrease traffic congestion, and lower emissions.
- What are the key factors driving the growth of the autonomous driving market?
- The growth of the autonomous driving market is driven by technological advancements, increasing demand for safer and more efficient transportation, and the growing interest from automotive and tech companies in developing new mobility solutions.
- How do visual-language models enhance autonomous driving?
- Visual-language models integrate visual data with natural language processing, enabling vehicles to better understand and respond to human commands and gestures. This enhances the user experience and makes autonomous driving more intuitive.