Weather prediction has long been a critical field, impacting everything from daily activities to large-scale disaster preparedness. Traditional methods, primarily based on Numerical Weather Prediction (NWP), have made significant strides but face limitations, especially with the increasing complexity of weather patterns and the vast amounts of data generated daily. This is where multimodal data comes into play, offering a transformative approach to weather prediction.
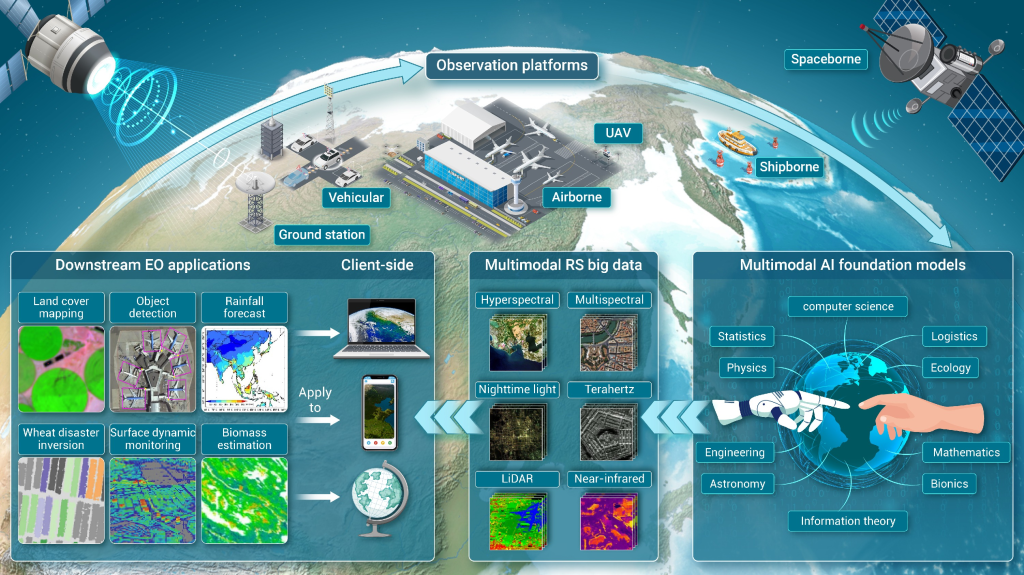
Multimodal data refers to the integration of various types of data, such as satellite imagery, radar data, temperature readings, humidity levels, and wind patterns. Unlike traditional single-modal approaches, multimodal data provides a more comprehensive and nuanced understanding of weather systems. For instance, while radar data can provide detailed information on precipitation, satellite imagery can offer insights into cloud formations and atmospheric conditions over large areas. By combining these different data sources, meteorologists can create more accurate and reliable weather forecasts.
The benefits of using multimodal data in weather prediction are manifold. One of the most significant advantages is the enhanced accuracy of forecasts. Traditional methods often struggle with the complexity and variability of weather systems, leading to inaccuracies and uncertainties. However, by integrating multiple data sources, models can capture a more complete picture of the atmosphere, leading to more precise predictions. For example, a study utilizing multimodal data for precipitation forecasting demonstrated a significant improvement in accuracy compared to single-modal approaches. This enhanced accuracy is crucial for various sectors, including agriculture, aviation, and disaster management, where reliable weather forecasts can make a substantial difference.
Another key benefit of multimodal data is improved computational efficiency. Traditional NWP methods require extensive computational resources and time to produce accurate forecasts. In contrast, deep learning models that leverage multimodal data can process large volumes of information more quickly and efficiently. These models can learn complex patterns and relationships within the data, allowing for faster and more accurate predictions. This efficiency is particularly important in the context of real-time weather forecasting, where timely and accurate information can be critical for decision-making.
Multimodal data also enables better handling of complex weather phenomena. Weather systems are inherently complex, involving multiple variables and interactions that can be difficult to model accurately. By integrating data from different sources, models can capture these complexities more effectively. For instance, the integration of spatial and temporal data allows for a more accurate representation of weather patterns and their evolution over time. This is particularly useful for predicting extreme weather events, such as hurricanes, tornadoes, and heavy rainfall, which can have significant impacts on human life and infrastructure.
Looking to the future, the use of multimodal data in weather prediction holds great promise. Advances in AI and machine learning are continually driving improvements in data processing and analysis, making it possible to extract even more valuable insights from multimodal data. This could lead to further enhancements in forecast accuracy and the ability to predict weather patterns with greater precision. Additionally, the potential for real-time forecasting and improved disaster preparedness could have profound implications for public safety and resource management.
In conclusion, the integration of multimodal data in weather prediction offers numerous benefits, including enhanced accuracy, improved computational efficiency, and better handling of complex weather phenomena. As technology continues to advance, the potential for further improvements in weather forecasting through the use of multimodal data is immense. This approach holds the promise of transforming weather prediction, making it more accurate, reliable, and timely, ultimately benefiting a wide range of sectors and improving our ability to prepare for and respond to weather-related challenges.
Recent Questions and Answers
- What types of multimodal data are commonly used in weather prediction?
- Common types include satellite imagery, radar data, temperature readings, humidity levels, and wind patterns.
- How does multimodal data improve the accuracy of weather forecasts compared to traditional methods?
- By providing a more comprehensive view of weather systems through the integration of multiple data sources, leading to more precise predictions.
- What are the computational advantages of using multimodal data in weather prediction?
- Multimodal data can be processed more efficiently by deep learning models, reducing the computational time and resources required compared to traditional NWP methods.
- Can multimodal data help in predicting extreme weather events more accurately?
- Yes, the integration of spatial and temporal data from multiple sources allows for a better representation of complex weather patterns, improving the accuracy of predictions for extreme events.
- What role do neural networks play in processing multimodal data for weather prediction?
- Neural networks, particularly deep learning models, can learn complex patterns and relationships within multimodal data, enabling faster and more accurate weather forecasts.
- What are the future prospects for the use of multimodal data in weather prediction?
- Advances in AI and machine learning are expected to further enhance the capabilities of multimodal data in weather prediction, potentially leading to real-time forecasting and improved disaster preparedness.
References
- DFMM-Precip: Deep Fusion of Multi-Modal Data for Precipitation Forecasting
- Multi-modal spatio-temporal meteorological forecasting
- Data-Driven Weather Forecasting and Climate Modeling